Tensorflow, Keras, Pytorch, Fast.ai 가 차례로 등장하며 딥러닝 개발 프레임워크의 전성기를 구가했다. 최근 5년동안 Google 추세를 살펴보자.
코드
library(gtrendsR)extrafont::loadfonts()result<-gtrends(keyword =c("pytorch","fastai", "tensorflow", "keras"), geo ="", time="today+5-y", low_search_volume =TRUE)gtrends_framework_g<-result$interest_over_time%>%as_tibble()%>%mutate(keyword =factor(keyword, levels =c("keras", "pytorch", "tensorflow", "fastai")))%>%mutate(hits =parse_number(hits))%>%ggplot(aes(x =date, y =hits, color =keyword))+geom_line()+theme_bw(base_family ="NanumBarunpen")+labs(x ="", y ="검색수", color ="프레임워크", title ="딥러닝 프레임워크 구글 검색 추세")+theme(legend.title =element_text(size =16), legend.text =element_text(size =14))# ragg always works for macragg::agg_png("images/dl_framework.png", width =297, height =210, units ="mm", res =300)gtrends_framework_gdev.off()
8.2 chatGPT 출현
chatGPT 출현이후 Tensorflow, Keras, Pytorch, Fast.ai 는 어떻게 전개될 것인지 최근 1년동안 Google 추세를 살펴보자.
코드
chatGPT_result<-gtrends(keyword =c("pytorch","fastai", "tensorflow", "keras", "chatGPT"), geo ="", time="today 12-m", low_search_volume =TRUE)gtrends_chatGPT_g<-chatGPT_result$interest_over_time%>%as_tibble()%>%mutate(keyword =factor(keyword, levels =c("chatGPT", "keras", "pytorch", "tensorflow", "fastai")))%>%mutate(hits =parse_number(hits))%>%mutate(date =as.Date(date))%>%ggplot(aes(x =date, y =hits, color =keyword))+geom_line()+theme_bw(base_family ="NanumBarunpen")+labs(x ="", y ="검색수", color ="프레임워크", title ="chatGPT와 딥러닝 프레임워크 구글 검색 추세")+scale_x_date(date_labels ="%Y-%m")+theme(legend.title =element_text(size =16), legend.text =element_text(size =14))# ragg always works for macragg::agg_png("images/chatGPT_framework.png", width =297, height =210, units ="mm", res =300)gtrends_chatGPT_gdev.off()
8.3 파이썬과 chatGPT
chatGPT 출현이후 파이썬, tensorflow, pytorch 최근 1년동안 Google 추세를 살펴보자.
코드
python_result<-gtrends(keyword =c("chatGPT", "pytorch","python", "tensorflow", "keras"), geo ="", time="today 12-m", low_search_volume =TRUE)python_chatGPT_g<-python_result$interest_over_time%>%as_tibble()%>%mutate(keyword =factor(keyword, levels =c("chatGPT", "python", "keras", "pytorch", "tensorflow")))%>%mutate(hits =parse_number(hits))%>%mutate(date =as.Date(date))%>%ggplot(aes(x =date, y =hits, color =keyword))+geom_line()+theme_bw(base_family ="NanumBarunpen")+labs(x ="", y ="검색수", color ="프레임워크", title ="파이썬, chatGPT, 주요 딥러닝 프레임워크 구글 검색 추세")+scale_x_date(date_labels ="%Y-%m")+theme(legend.title =element_text(size =16), legend.text =element_text(size =14))# ragg always works for macragg::agg_png("images/python_chatGPT_g.png", width =297, height =210, units ="mm", res =300)python_chatGPT_gdev.off()
소스 코드
---title: "chatGPT"subtitle: "프로젝트"author: - name: 이광춘 url: https://www.linkedin.com/in/kwangchunlee/ affiliation: 한국 R 사용자회 affiliation-url: https://github.com/bit2rtitle-block-banner: true#title-block-banner: "#562457"format: html: css: css/quarto.css theme: flatly code-fold: true toc: true toc-depth: 3 toc-title: 목차 number-sections: true highlight-style: github self-contained: falsefilters: - lightboxlightbox: autolink-citations: yesknitr: opts_chunk: message: false warning: false collapse: true comment: "#>" R.options: knitr.graphics.auto_pdf: trueeditor_options: chunk_output_type: console---# 1 백만 사용자 {{< fa solid rocket >}}1 백만 가입자를 가질 때까지 걸린 소요시간을 보면 chatGPT 의 영향력을 파악할 수 있다.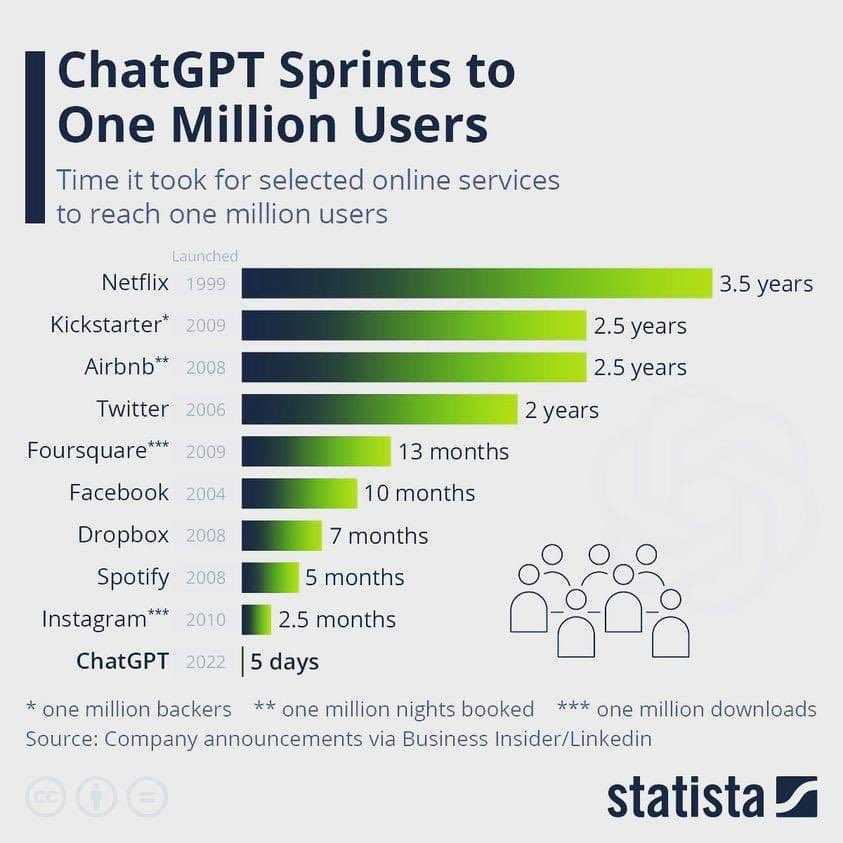# 패러다임[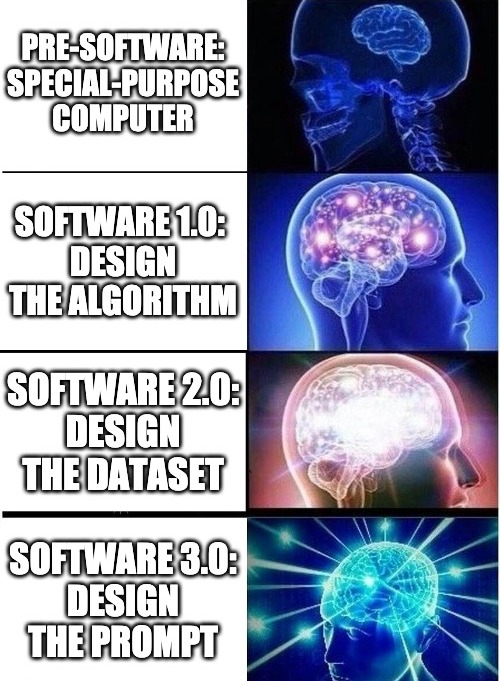]{.aside}[- Pre-Software: Special-purpose computer <br>- Software 1.0: Design the Algorithm <br>- Software 2.0: Design the Dataset <br>- Software 3.0: Design the Prompt]{.aside}:::{.panel-tabset .column-page}## Feature Engineering- **패러다임**: (비신경망)지도학습 (Fully Supervised Learning)- **전성기**: 2015년까지 최고 전성기 구가- **특징** 1. 주로 비신경망 기계학습이 사용 1. 수작업으로 Feature를 추출- **대표작** 1. 수작업 Feature 추출 후 SVM(support vector machine) 기계학습 모형 1. 수작업 Feature 추출 후 CRF(conditional random fields)## Architecture Engineering- **패러다임**: 신경망 지도학습(Fully Supervised Learning)- **전성기**: 대략 2013~2018- **특징** 1. 신경망(Neural Network) 의존 1. 수작업으로 Feature를 손볼 필요는 없으나 신경망 네트워크는 수정해야 함(LSTM vs CNN) 1. 종종 사전학습된 언어모형을 사용하나 임베딩(embedding) 같은 얕은(shallow) Feature를 적용- **대표작** 1. 텍스트 분류작업에 CNN 사용## Objective Engineering- **패러다임**: 사전학습(pre-training), 미세조정(fine-tuning)- **전성기**: 2017~현재- **특징** 1. 사전학습된 언어모형을 전체 모형의 초기값으로 사용 1. 아키텍쳐 디자인에 작업이 덜 필요하지만 목적함수(Objective function) 엔지니어링은 필요- **대표작** 1. BERT → Fine Tuning## Prompt Engineering- **패러다임**: 사전학습(pre-training), 프롬프트(Prompt), 예측(Predict)- **전성기**: 2019~현재- **특징** 1. NLP 작업이 언어모형(Language Model)에 전적으로 의존 1. 얕던 깊던 Feature 추출, 예측 등 작업이 전적으로 언어모형에 의존 1. 프롬프트 공학이 필요- **대표작** 1. GPT3:::# Prompt engineering- Instructions- Question- Input data- Examples[[Xavier (Xavi) Amatriain(January 5, 2023), "Prompt Engineering 101 - Introduction and resources", Linkedin](https://www.linkedin.com/pulse/prompt-engineering-101-introduction-resources-amatriain/),[Prompt Engineering - Learn how to use AI models with prompt engineering](https://microsoft.github.io/prompt-engineering/)]{.aside}# Genearative AI- 구분 - generation: text → image - classification: image → text - transformation: image → image (or text → text)- AI 프로젝트 - GPT-3 - Dalle.2 (text-to-image) - Meta’s AI (text-to-video) - Google AI (text-to-video) - Stable Diffusion (text-to-image) - Tesla AI (humanoid robot + self-driving)- text-to-X - text-to-gif (T2G) - text-to-3D (T2D) - text-to-text (T2T) - text-to-NFT (T2N) - text-to-code (T2C) - text-to-image (T2I) - text-to-audio (T2S) - text-to-video (T2V) - text-to-music (T2M) - text-to-motion (T2Mo)- 기타 - brain-to-text (B2T) - image-to-text (I2T) - speech-to-text (S2T) - audio-to-audio (A2A) - tweet-to-image (Tt2I) - text-to-sound (T2S)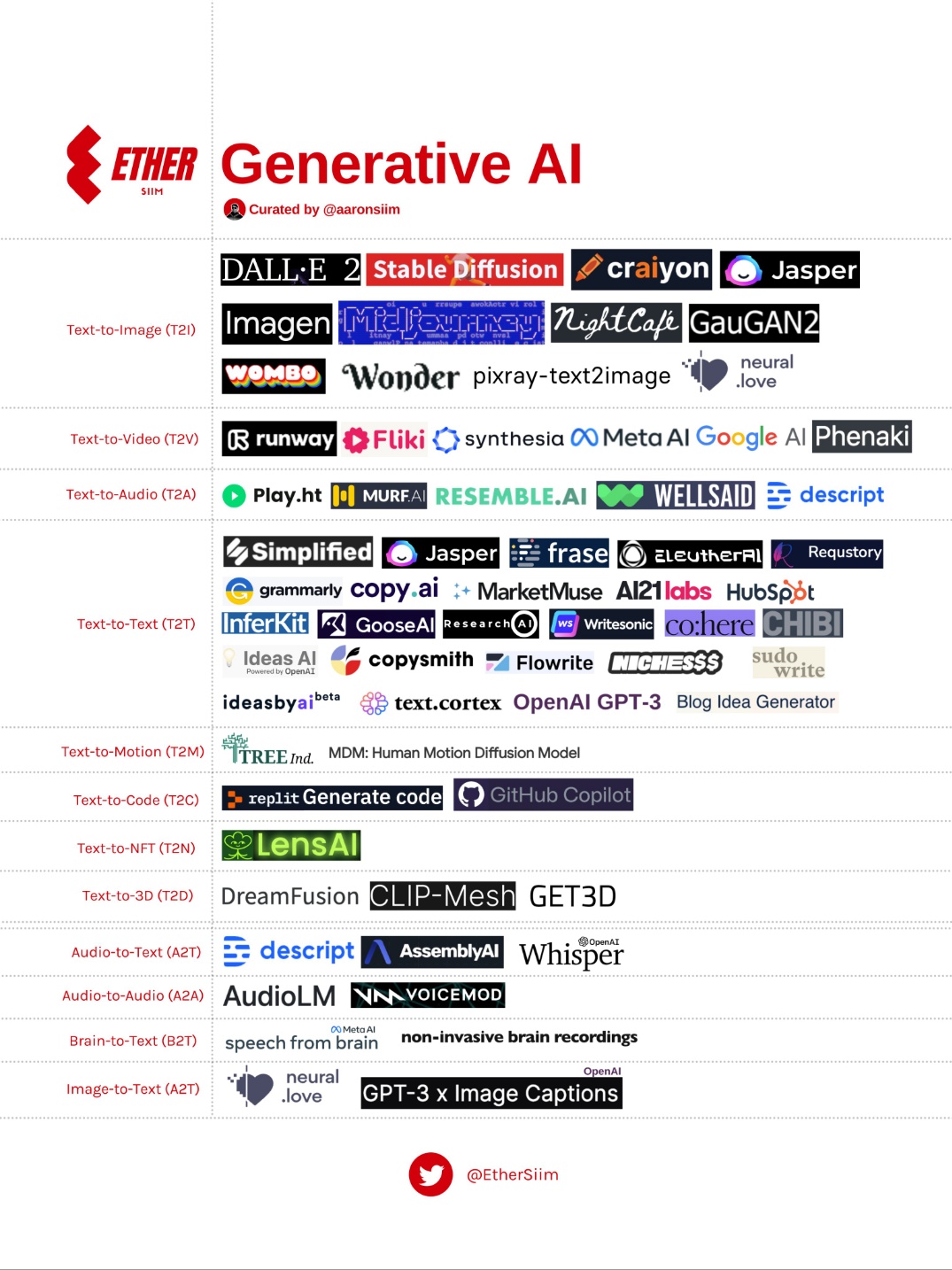# 데이터와 하드웨어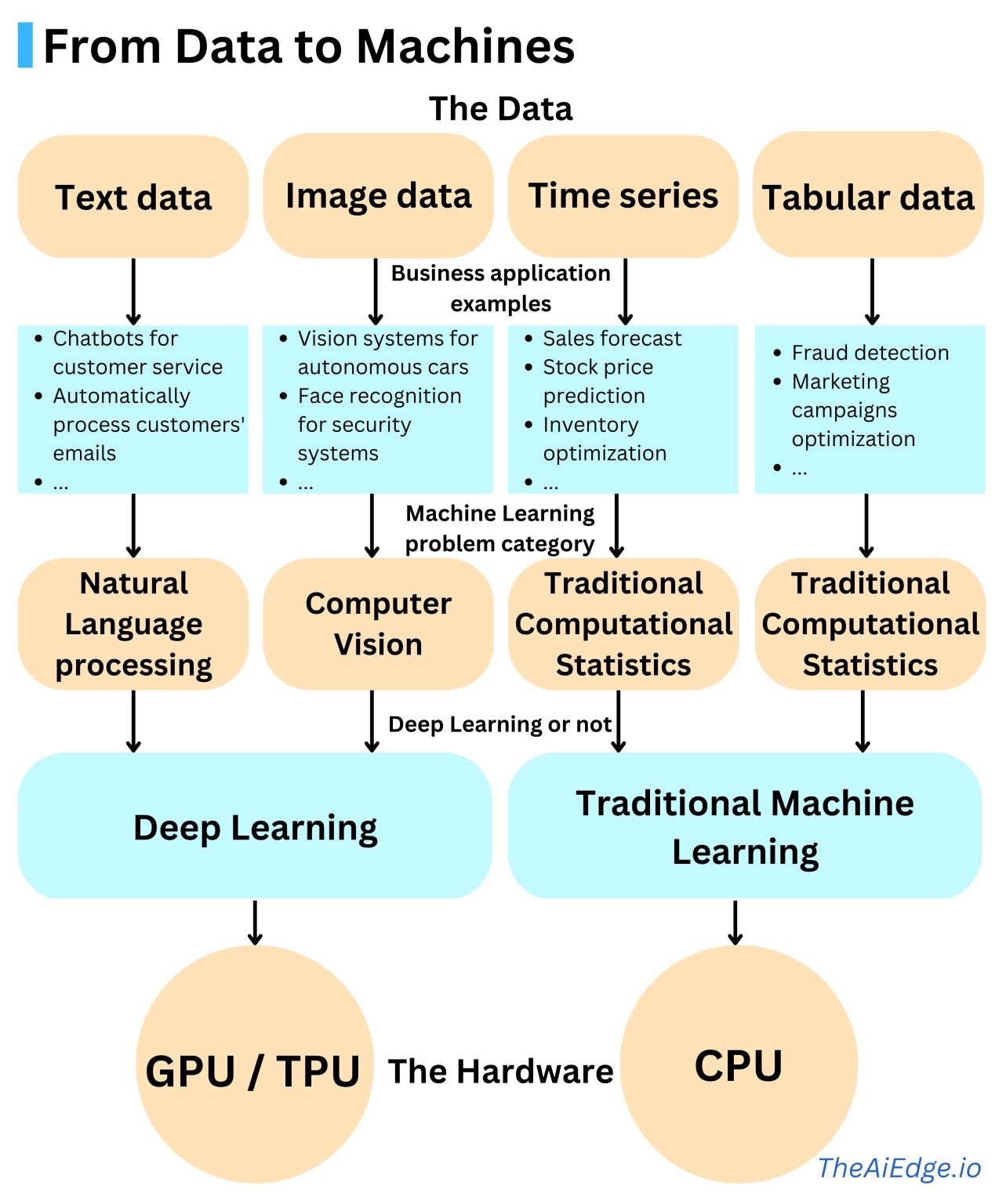# Open Assistant::: {#fig-assistant layout-ncol=3}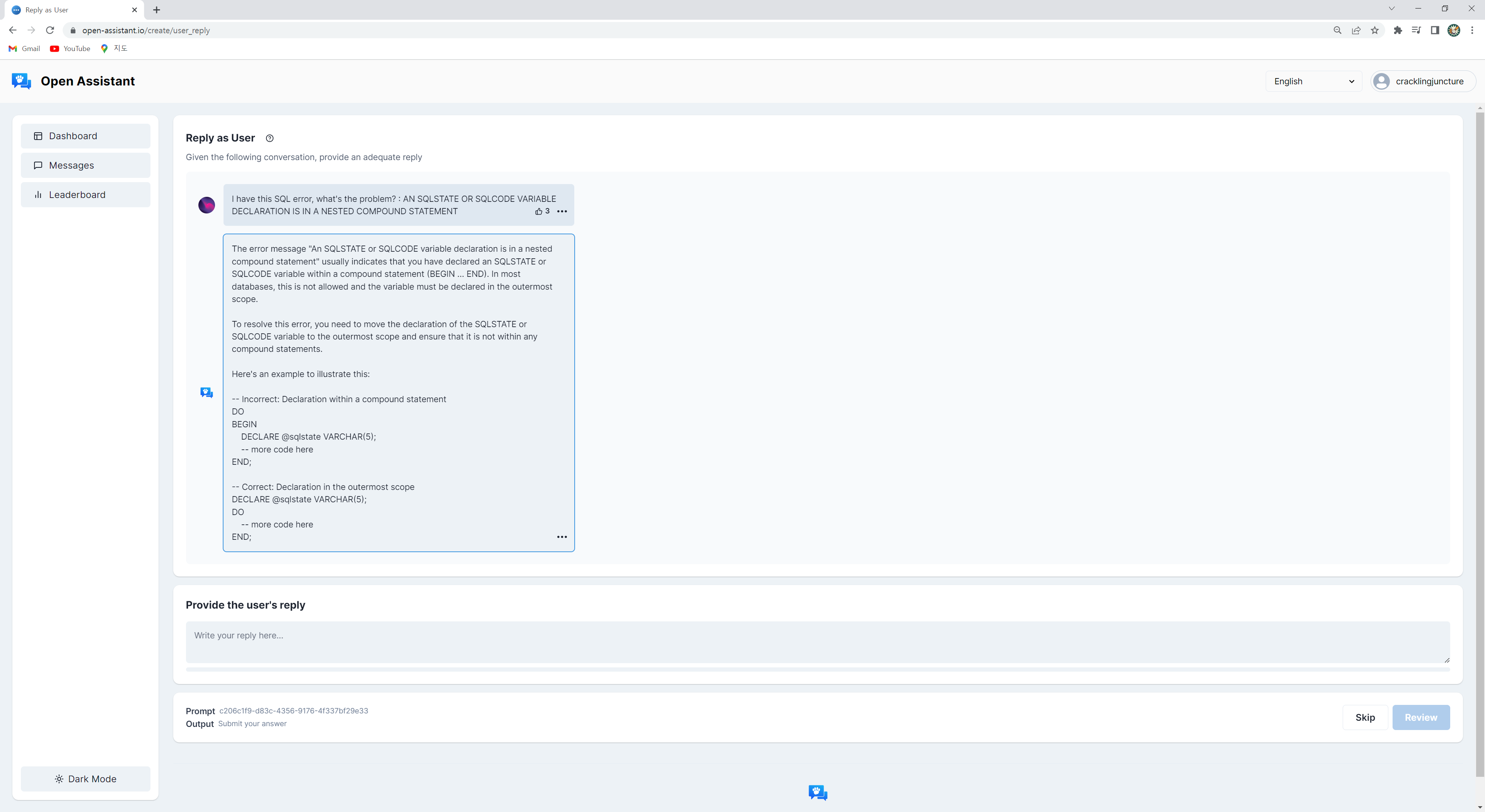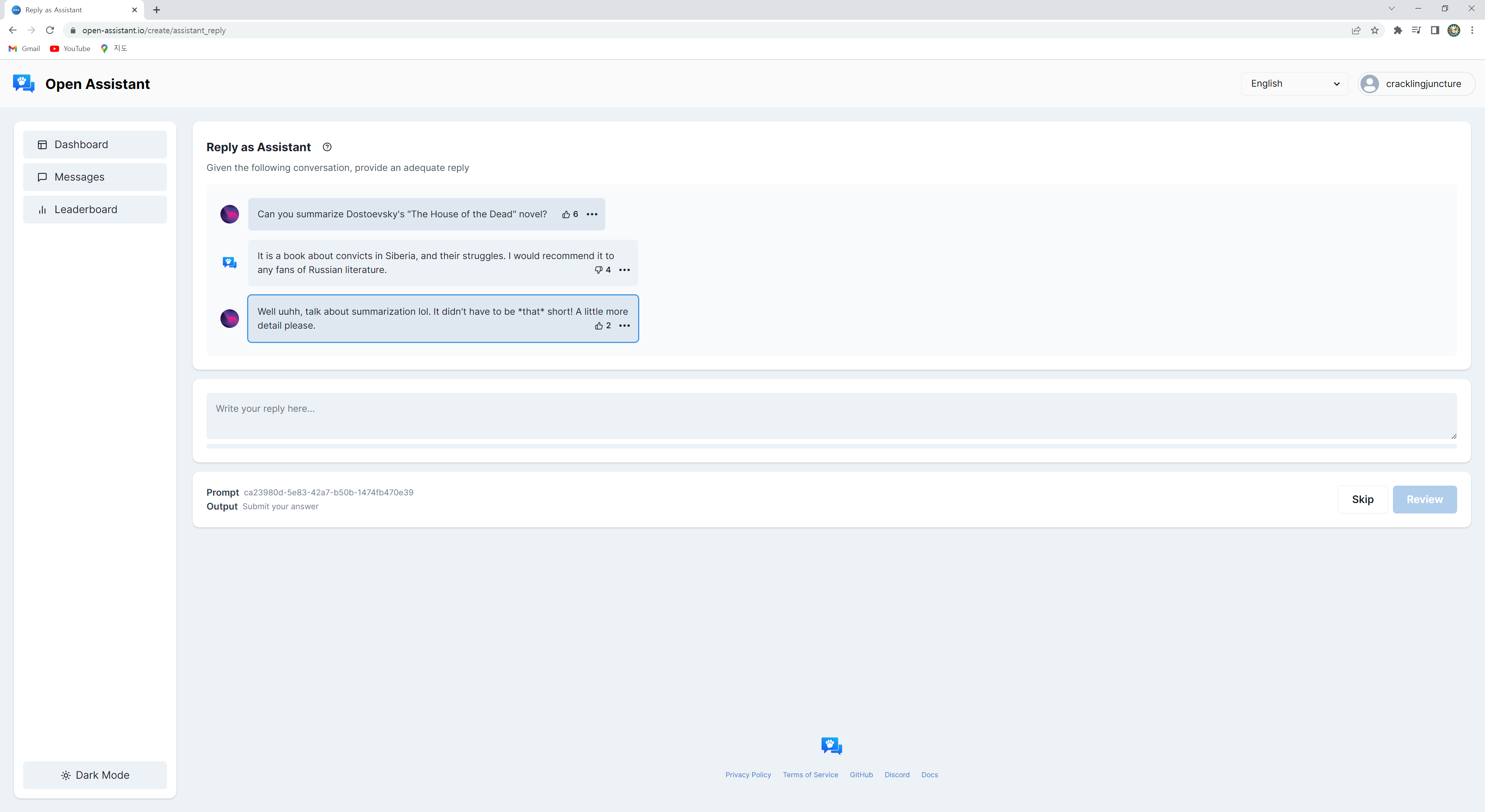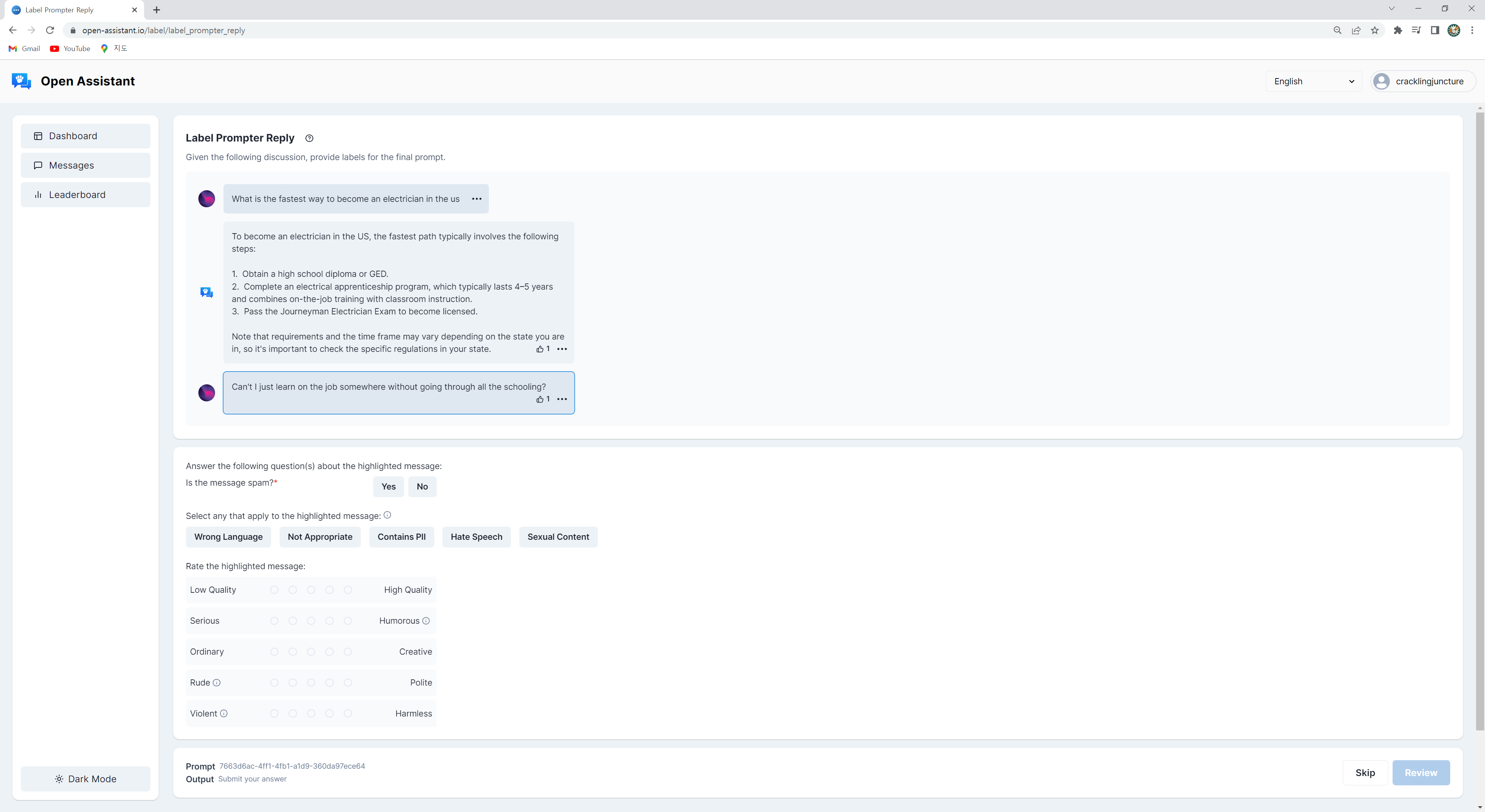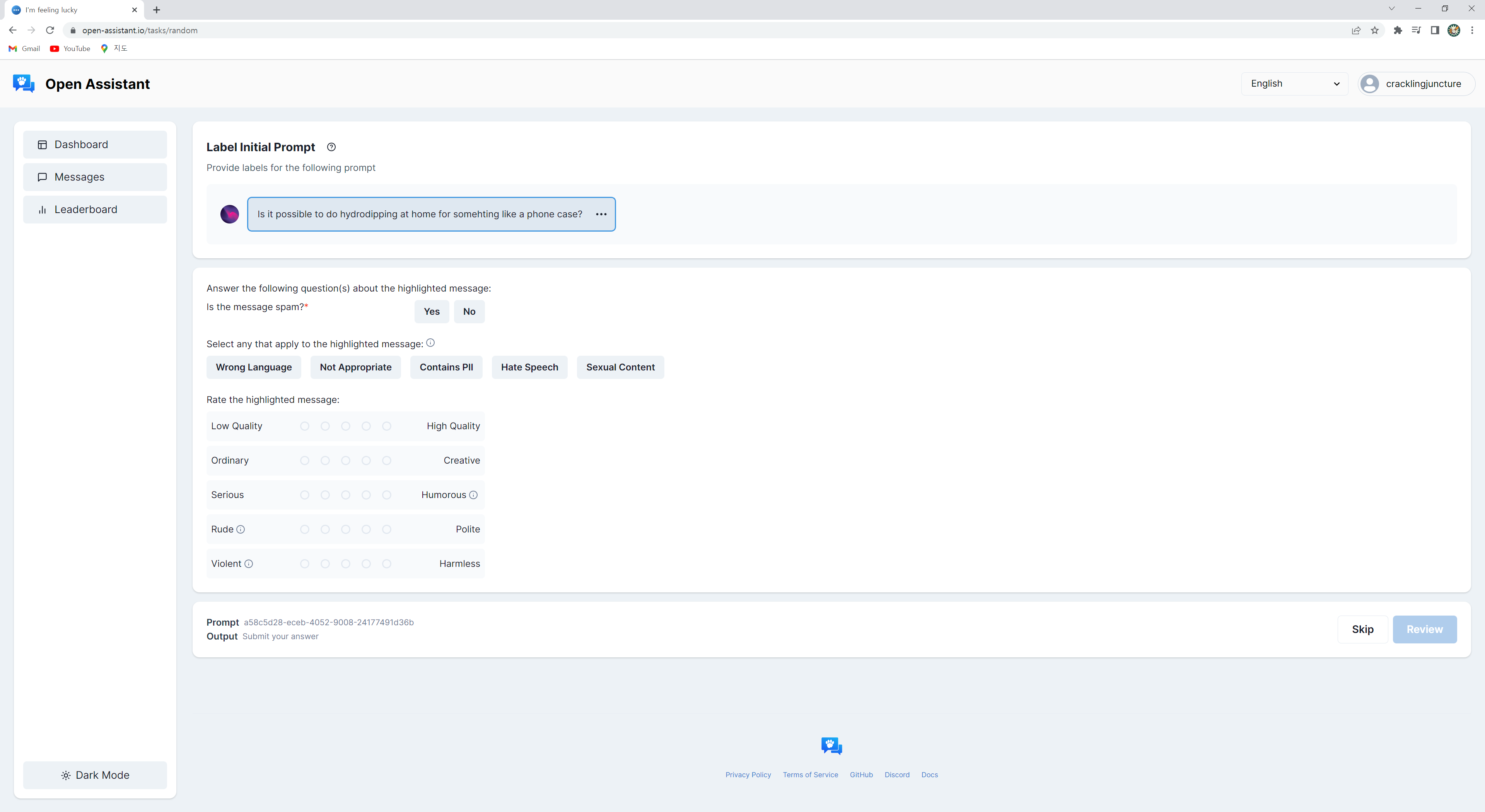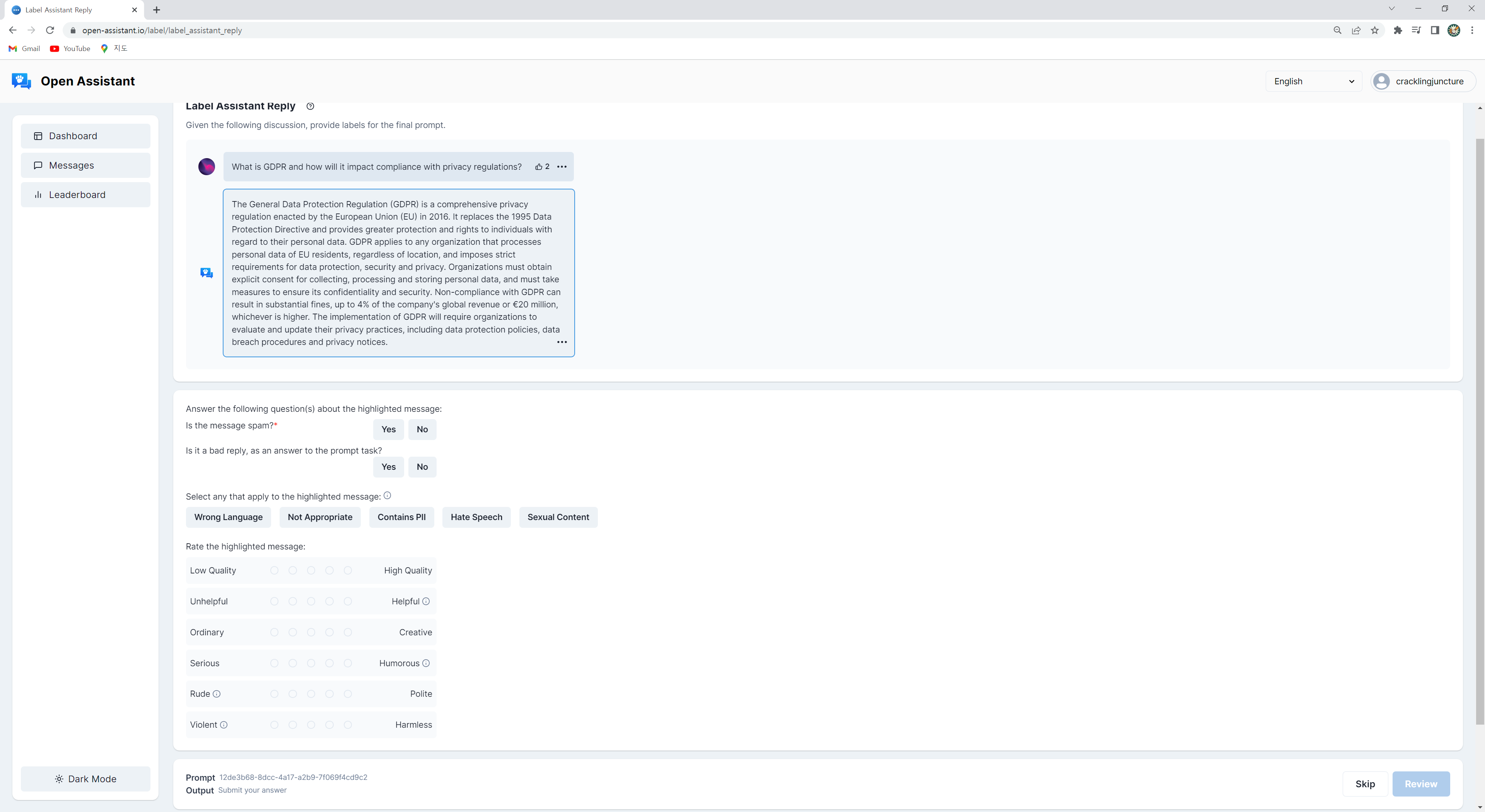[Open Assistant](https://open-assistant.io/dashboard):::# GPT-3 언어 데이터GPT-3 개발에 투입된 문서갯수를 언어별로 살펴보자.```{r}#| eval: falselibrary(tidyverse)library(gt)library(countrycode)library(rvest)library(gtExtras)## 언어 코드 lang_tbl <-read_html(x ='http://www.lingoes.net/en/translator/langcode.htm') %>%html_element(css ='body > table') %>%html_table() %>%set_names(c("언어", "언어명"))gpt_raw <-read_csv("https://raw.githubusercontent.com/openai/gpt-3/master/dataset_statistics/languages_by_document_count.csv")gpt_tbl <- gpt_raw %>%set_names(c("언어", "문서수", "비중")) %>%mutate(비중 =parse_number(비중) /100) %>%mutate(누적문서 =cumsum(문서수)) %>%mutate(누적비중 = 누적문서 /sum(문서수)) %>%top_n(문서수, n =28) gpt_gt <- gpt_tbl %>%left_join(lang_tbl, by ="언어") %>%select(언어, 언어명, 문서수, 비중, 누적비중) %>%## 표 gt() %>%gt_theme_nytimes() %>%tab_header(title =md("**GPT-3 언어모형 개발에 사용된 언어별 문서 통계**"),subtitle ="한국어 포함 상위 28개 언어") %>%tab_source_note(source_note ="자료출처: https://github.com/openai/gpt-3/blob/master/dataset_statistics/languages_by_document_count.csv") %>%tab_spanner(label ="언어코드와 언어명",columns =c(언어, 언어명)) %>%tab_spanner(label ="통계수치",columns =c(문서수, 비중, 누적비중)) %>%cols_align(align ="center",columns =c(언어, 언어명)) %>%# tab_style(# style = cell_text(size = px(12)),# locations = cells_body(# columns = c(문서수, 비중, 누적비중)# )# ) %>% fmt_percent(columns =c(비중, 누적비중),decimals =2 ) %>%fmt_number(columns = 문서수,decimals =0,sep_mark ="," ) %>%gt_highlight_rows(rows =c(1,28),fill ="lightgrey",target_col = 언어 ) %>%sub_missing(columns =everything(),missing_text ="-" ) gpt_gt %>%gtsave("images/gpt_lang.png")```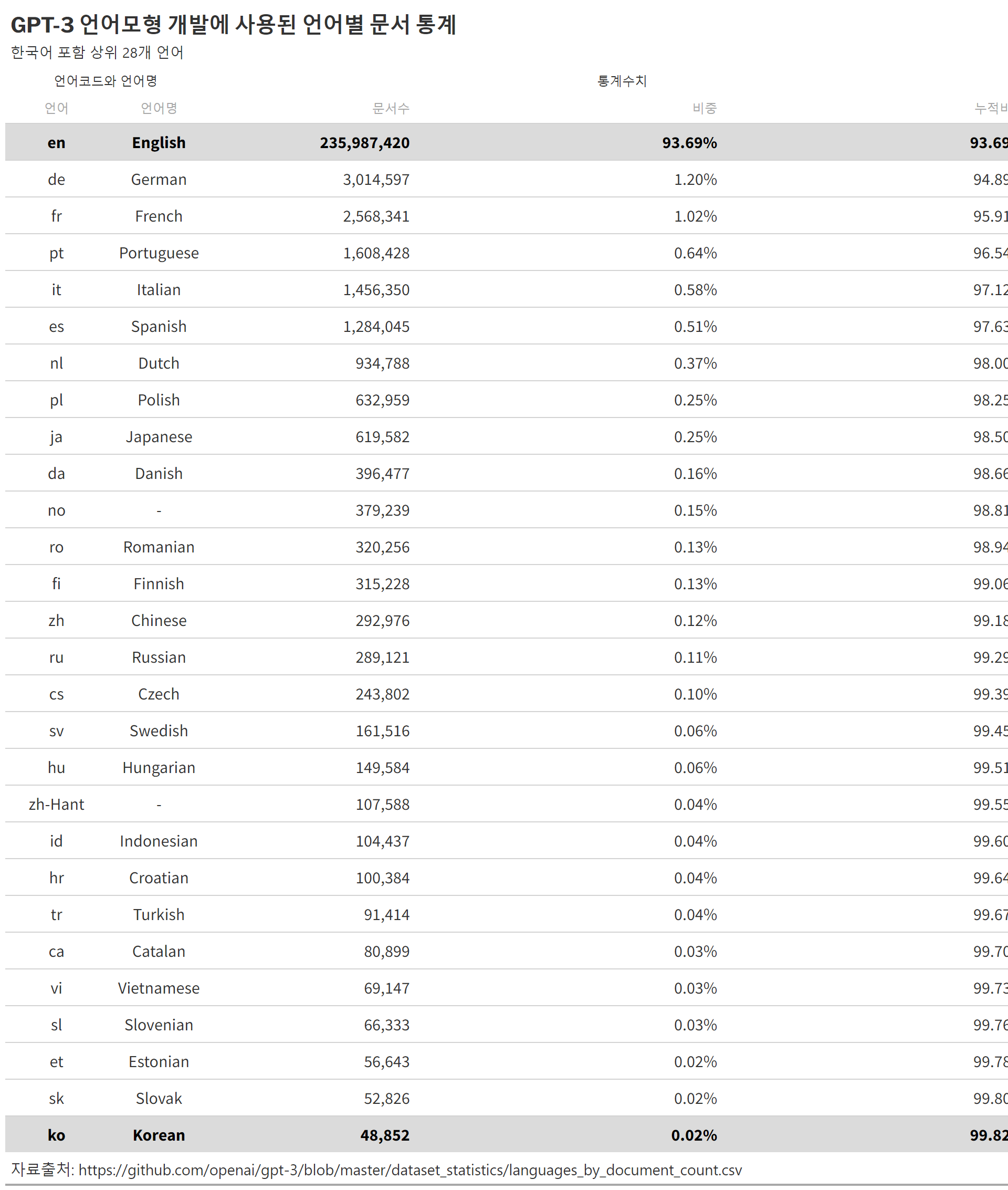# 구글 트렌드## chatGPT 이전Tensorflow, Keras, Pytorch, Fast.ai 가 차례로 등장하며 딥러닝 개발 프레임워크의 전성기를 구가했다.최근 5년동안 Google 추세를 살펴보자.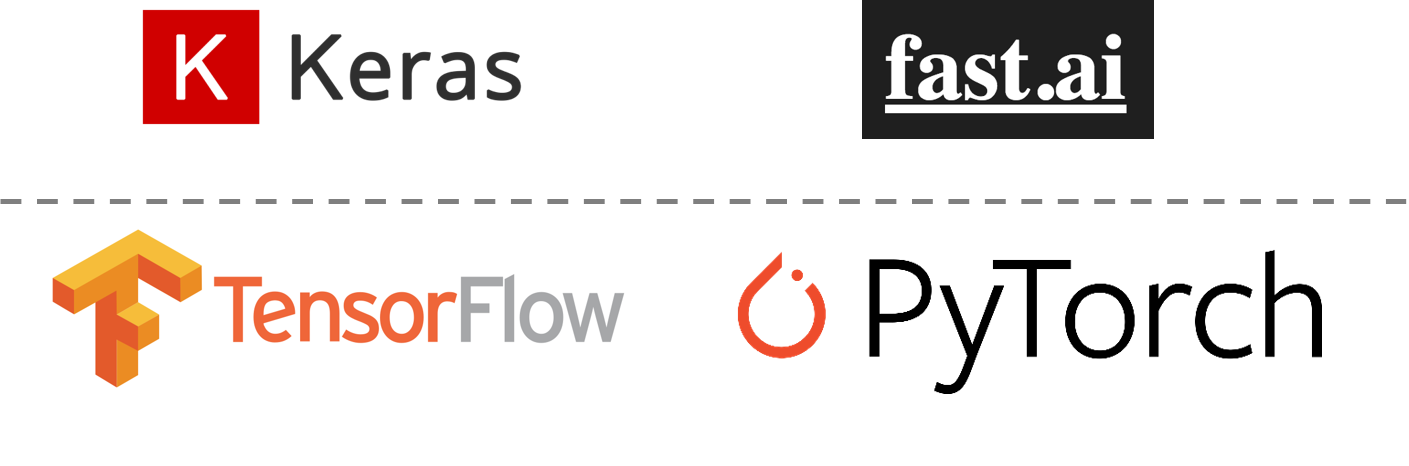```{r}#| eval: falselibrary(gtrendsR)extrafont::loadfonts()result <-gtrends(keyword =c("pytorch","fastai", "tensorflow", "keras"), geo ="", time="today+5-y", low_search_volume =TRUE)gtrends_framework_g <- result$interest_over_time %>%as_tibble() %>%mutate(keyword =factor(keyword, levels =c("keras", "pytorch", "tensorflow", "fastai"))) %>%mutate(hits =parse_number(hits)) %>%ggplot(aes(x = date, y = hits, color = keyword)) +geom_line() +theme_bw(base_family ="NanumBarunpen") +labs(x ="", y ="검색수",color ="프레임워크",title ="딥러닝 프레임워크 구글 검색 추세") +theme(legend.title =element_text(size =16),legend.text =element_text(size =14))# ragg always works for macragg::agg_png("images/dl_framework.png", width =297, height =210, units ="mm", res =300)gtrends_framework_gdev.off()```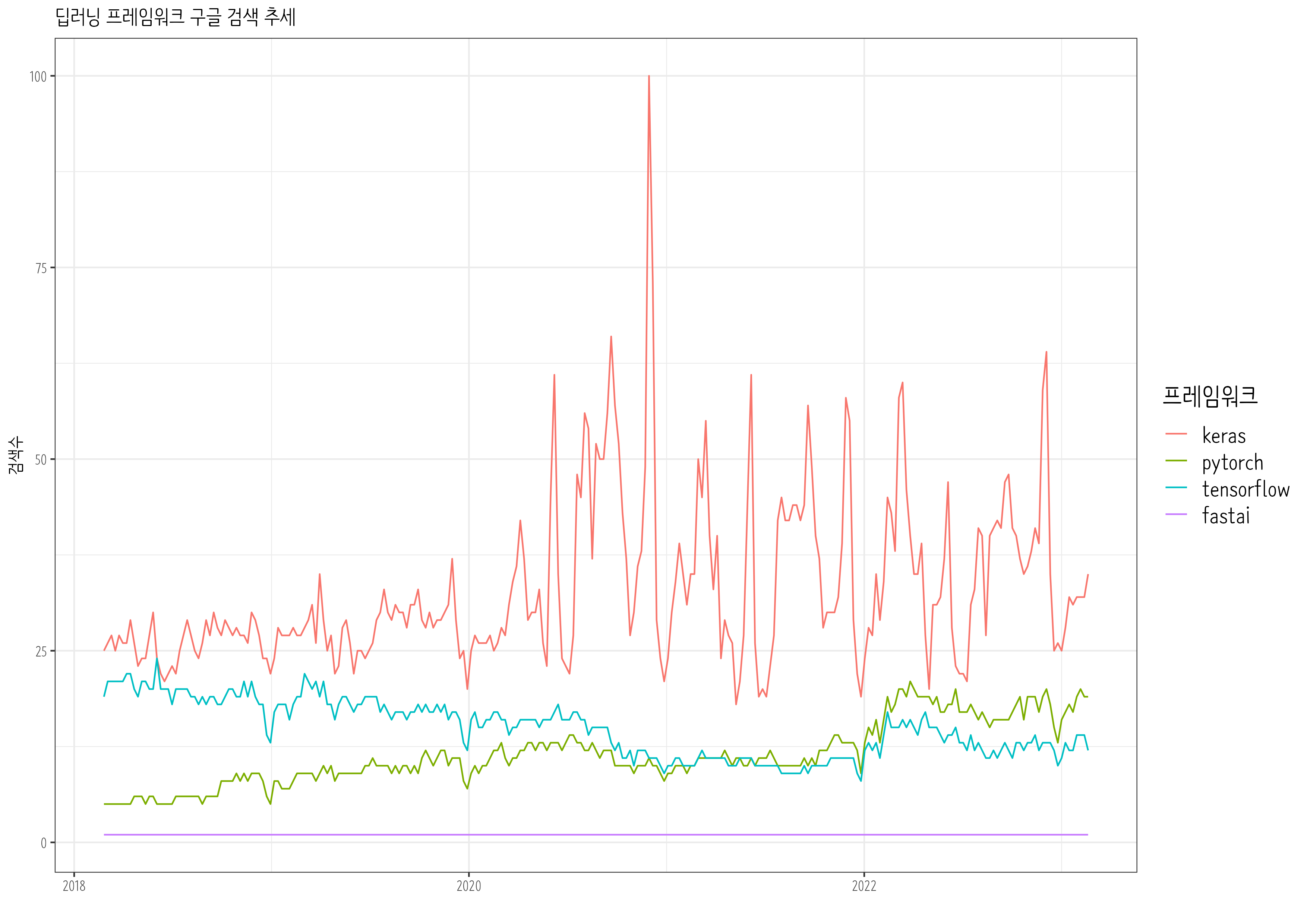## chatGPT 출현chatGPT 출현이후 Tensorflow, Keras, Pytorch, Fast.ai 는 어떻게 전개될 것인지최근 1년동안 Google 추세를 살펴보자.```{r}#| eval: falsechatGPT_result <-gtrends(keyword =c("pytorch","fastai", "tensorflow", "keras", "chatGPT"), geo ="", time="today 12-m", low_search_volume =TRUE)gtrends_chatGPT_g <- chatGPT_result$interest_over_time %>%as_tibble() %>%mutate(keyword =factor(keyword, levels =c("chatGPT", "keras", "pytorch", "tensorflow", "fastai"))) %>%mutate(hits =parse_number(hits)) %>%mutate(date =as.Date(date)) %>%ggplot(aes(x = date, y = hits, color = keyword)) +geom_line() +theme_bw(base_family ="NanumBarunpen") +labs(x ="", y ="검색수",color ="프레임워크",title ="chatGPT와 딥러닝 프레임워크 구글 검색 추세") +scale_x_date(date_labels ="%Y-%m") +theme(legend.title =element_text(size =16),legend.text =element_text(size =14))# ragg always works for macragg::agg_png("images/chatGPT_framework.png", width =297, height =210, units ="mm", res =300)gtrends_chatGPT_gdev.off()```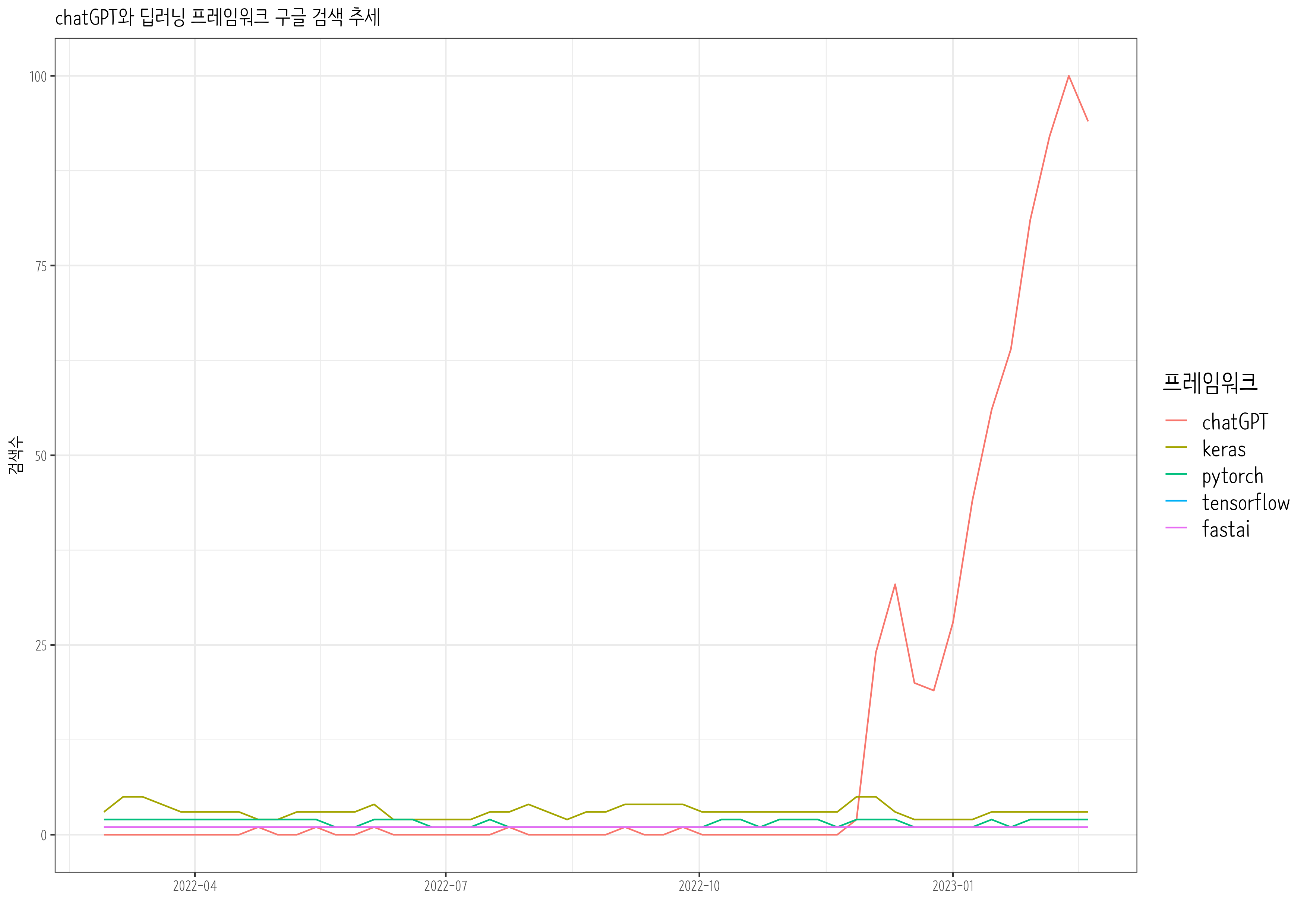## 파이썬과 chatGPTchatGPT 출현이후 파이썬, tensorflow, pytorch 최근 1년동안 Google 추세를 살펴보자.```{r}#| eval: falsepython_result <-gtrends(keyword =c("chatGPT", "pytorch","python", "tensorflow", "keras"), geo ="", time="today 12-m", low_search_volume =TRUE)python_chatGPT_g <- python_result$interest_over_time %>%as_tibble() %>%mutate(keyword =factor(keyword, levels =c("chatGPT", "python", "keras", "pytorch", "tensorflow"))) %>%mutate(hits =parse_number(hits)) %>%mutate(date =as.Date(date)) %>%ggplot(aes(x = date, y = hits, color = keyword)) +geom_line() +theme_bw(base_family ="NanumBarunpen") +labs(x ="", y ="검색수",color ="프레임워크",title ="파이썬, chatGPT, 주요 딥러닝 프레임워크 구글 검색 추세") +scale_x_date(date_labels ="%Y-%m") +theme(legend.title =element_text(size =16),legend.text =element_text(size =14))# ragg always works for macragg::agg_png("images/python_chatGPT_g.png", width =297, height =210, units ="mm", res =300)python_chatGPT_gdev.off()```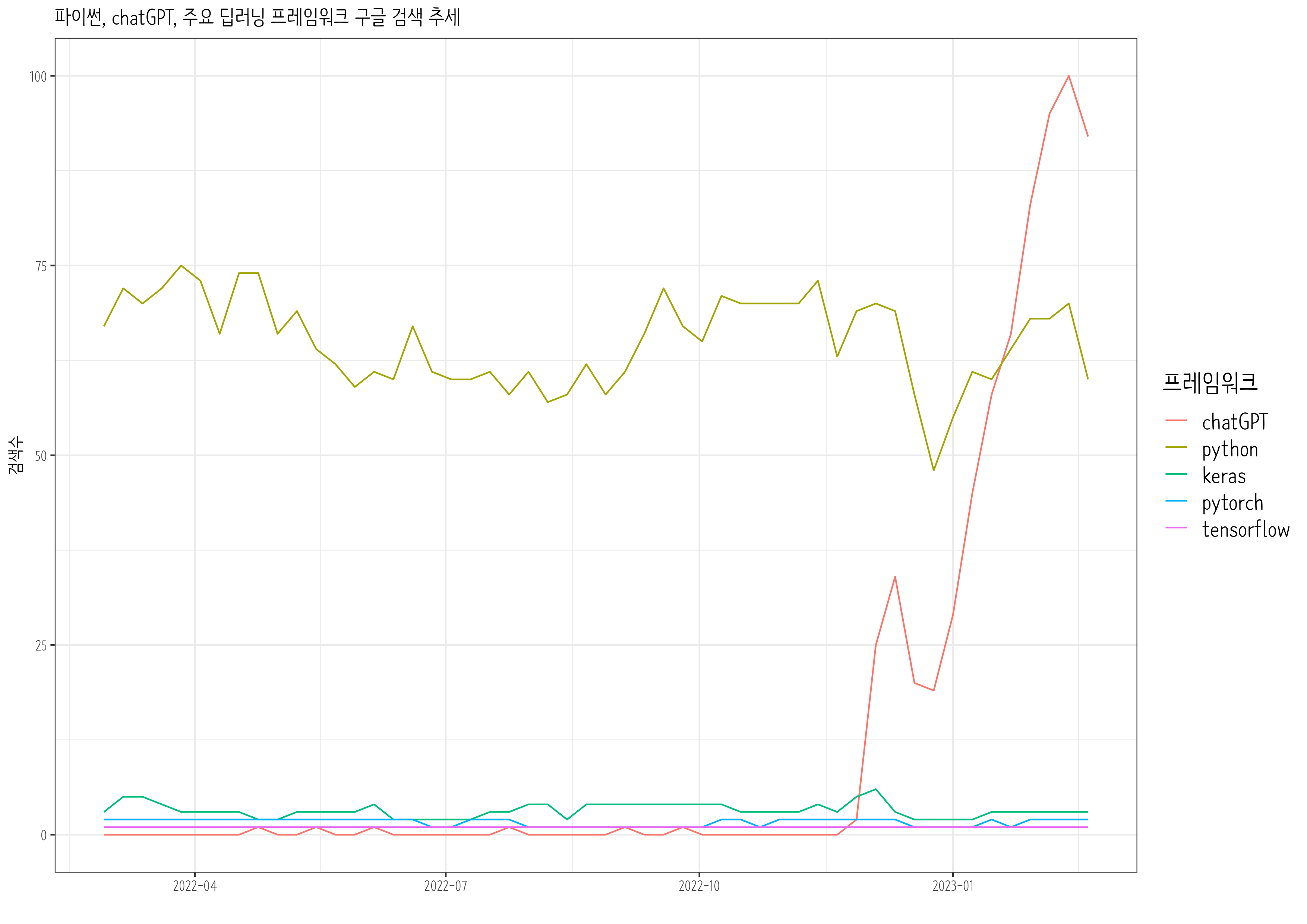